Revolutionising Image Compression: AI Enhancing WebP and JPEG with Human-Eye Perception
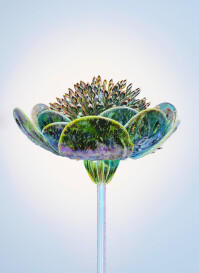
Digital media is of utmost importance in today's world, impacting communication, entertainment, marketing, and information sharing. Smaller image files play a crucial role in this landscape. They ensure faster loading times, enhancing user experience on websites and applications. With reduced file sizes, bandwidth consumption is minimised, benefiting users with limited connectivity and reducing costs. Moreover, smaller image files optimise storage space, benefiting cloud storage, content management systems, and e-commerce platforms. In summary, smaller image files are essential for improving user experience, optimising data usage, and enhancing storage efficiency in the digital media realm.
Digital Media, Accessibility and AI
Our journey with digital media enhancements begins with incorporating accessibility into the digital media where accessibility content is interwoven with the image itself. We don’t mean a separation of accessibility content as metadata verses image content, but rather where accessibility content is in fact encoded into the image itself and is the image.
In many ways this is a form of steganography. Steganography is a technique used in digital media to hide secret information within seemingly innocuous files, such as images, audio, or video. It involves embedding the hidden data in a way that is imperceptible to the human eye or ear. Steganography ensures covert communication and can be used for various purposes, including data security and privacy.
At snapWONDERS, we were intrigued by the idea of using AI to assist in incorporating accessibility content into digital media and improving existing steganography technologies to increase the size of the information payload within the digital media itself.
Moreover, if AI were trained to comprehend the visual perception of digital content by the human eye in natural photos and identify areas where information loss is negligible compared to noticeable loss, we could potentially eliminate redundant aspects of image content when viewing such photos.
Our findings have found that 30% to 50% of image content in natural photo images is redundant.
Original image:
Image with 30.3% information content stripped out:
AI and the big picture
What do you observe in the image above? Do you notice the similarities in colour and contrast? Or perhaps you perceive the arrangement of different letters in a stack? Furthermore, when you view the image as a whole, what impressions does it evoke?
The reality is the human mind possesses the remarkable ability to perceive and analyse both the intricate details of an image and simultaneously comprehend its overall contextual significance.
AI can help by looking at redundancies of information in the big picture.
An overview on JPEG and WebP
JPEG (Joint Photographic Experts Group) is a widely used image format developed in the 1990s, efficiently compressing photographic images while maintaining acceptable quality. It reduces file sizes without significant loss, making it popular. WebP, introduced by Google in 2010, addresses JPEG's limitations. WebP uses lossy and lossless compression, offering superior image quality at smaller sizes. It supports transparency and animation, making it versatile for web applications. Both formats provide efficient compression and visual quality, with WebP enhancing these benefits with advanced features.
The core logic behind JPEG is based on the fact that the human visual system is more sensitive to changes in brightness than changes in colour. To achieve compression, JPEG divides the image into blocks of pixels and applies a Discrete Cosine Transform (DCT) to convert the pixel values from the spatial domain to the frequency domain. It then quantises the transformed coefficients based on their visual importance and discards high-frequency components that are less perceptible to the human eye. Finally, the compressed image is encoded using variable-length coding, resulting in a smaller file size while maintaining an acceptable level of visual quality.
WebP, on the other hand, involves a combination of predictive coding, transform coding, and entropy coding. Predictive coding is used to predict the values of pixels based on their neighbouring pixels, reducing redundancy in the image data. Transform coding, similar to JPEG, applies a transform (in this case, the Discrete Cosine Transform or DCT) to convert the pixel values into a frequency domain representation. The transformed coefficients are then quantised and encoded using variable-length coding. Additionally, WebP employs advanced techniques such as spatial prediction and colour space conversion to further enhance compression efficiency. This combination of compression methods allows WebP to achieve smaller file sizes while maintaining good image quality.
AI enhancing JPEG and WebP
AI has the potential to enhance existing image compression techniques by taking into account the overall context of the natural photo. While neighbouring pixels are important, what truly matters is the context of the pixels within the entire picture. By intelligently analysing this context, AI can effectively reduce information content in certain areas more aggressively while preserving it in others. As a result, when the image is viewed as a whole, the perceived loss of information is minimal. This approach can lead to even greater levels of image compression.
However, one of the challenges when employing this method is that the information redundancies within the image is variable and there needs to be a way for AI to determine which regions of the image can be more aggressively reduced when compared to other regions without having to store this information itself.
This means the role of the AI would be twofold. The first is to identify areas for reduction in an image where information loss is negligible compared to noticeable loss, and the second is to determine the reverse when given the reduced image which is to be able to detail what the original information loss was.
snapWONDERS has achieved a significant milestone by harnessing the power of AI to solve the above two problems. This achievement marks a significant stride towards maximising the storage of accessibility payload data within images with minimal perceived visual image loss. We are thrilled to explore this topic further in our upcoming article, where we will demonstrate these concepts in more detail.
Stay tuned!
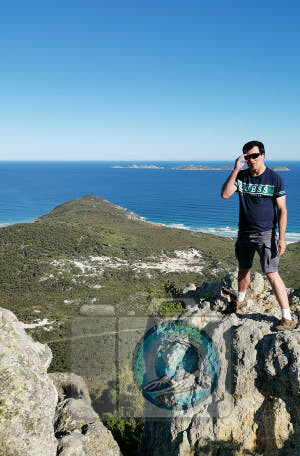